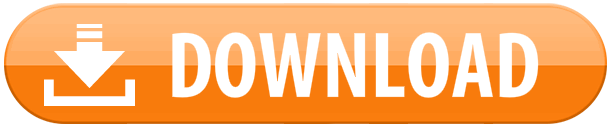

The genetic correlation reflects how much the traits are linked by genetic via polygenic effect or linkage desequilibrium, whereas the residual correlation reflects the environmental correlation or errors measurement correlation.Īlthough we can calculate this by hand, we can also use vpredict(), which also provides an (approximate) standard error: The additive genetic variance-covariance matrix ( \(\textbf\) = 0.4.īoth correlations are distinct in nature. Modela has fitted a bivariate model of bwt and tarsus, with the mean for each of the traits as a fixed effect ( trait). Modela <- update(modela) # Model fitted using the sigma parameterization. Notes that if the LogLik is not stabilized after several iterations, it is good indication of the model require more iteration.
Asreml r update#
Another way to increase the number of iteration will be to use the update function. Finally, we have increased the default maximum number of iterations ( maxiter) which can help to achieve convergence for more complicated models. It is also possible to let the model running without specifying starting values. These can be very approximate guestimates or not at all, but having reasonable starting values can help convergence. We can also specify some starting values for the variance matrices. We need to explicitly specify a covariance structure with difference covariance functions us(), idh() or corgh() which for example would estimate an unconstrained (co)variance matrix, an identity matrix and a variance and correlation matrix repestively. This is because ASReml-R allows us to make different assumptions about the way in which traits might be related.
Asreml r code#
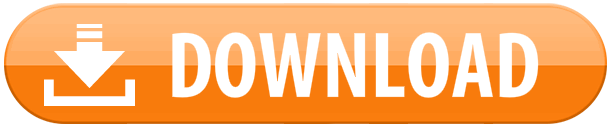